This feature is found in the modern Automate driving systems which are dependable on advance image classifications. Accuracy as well as detailed image annotations are critical factors for the training process of the machine learning models that enable the self-driving cars perception system. Here are ways in which advanced image labelling techniques contribute to improving autonomous vehicles:
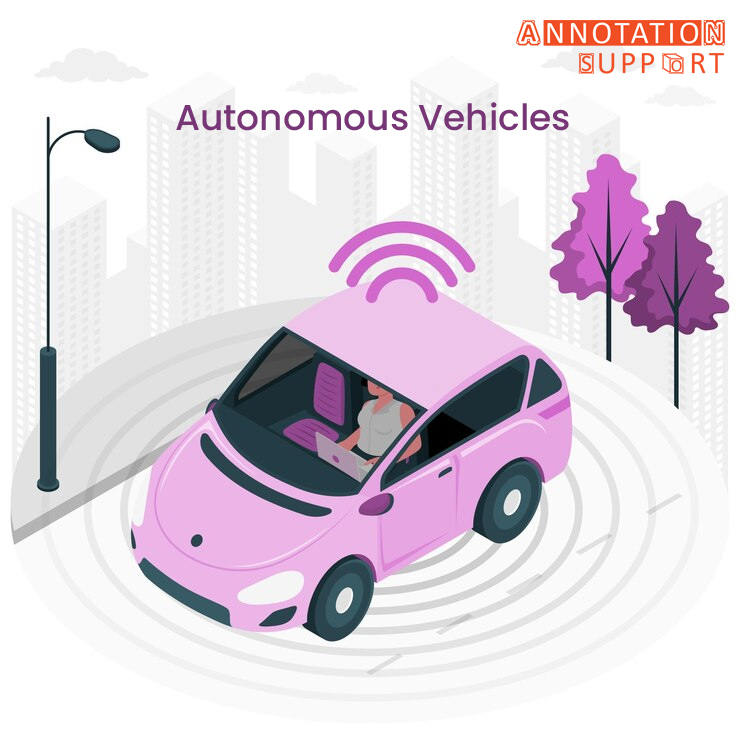
Here are ways in which advanced image labelling techniques contribute to improving autonomous vehicles:
Fine-Grained Object Detection:
- Through advanced annotation techniques that include, but not limited to, exact bounding boxes and polygon annotations, let machine learning modules detect objects with more precision and less ambiguity.
- The line-line annotations are a granular description of the shape and location of objects leading to the cars perception of its surroundings enhancement.
Semantic Segmentation:
- To supply semantic segmentation using the pixel-level identification, the model can now classify not merely each pixel but whole image.
- Such attributes facilitate the car in identifying the objects, and helps to understand the semantic meaning of the components of the scene.
Instance Segmentation:
- Use instance segmentation, which does object instances recognition and boundary delineation in a given scene.
- Particularly helpful in the cases where different objects of the same category are presented with the system to pick the right object for complicated and specific scenario.
Dynamic Object Tracking:
- Add interactive objects tracing annotations that can mark object paths through the picture.
- This is of primary importance for the on-board systems to evaluate the dynamics of obstacles and increase the safety and integrity of automation in decision-making.
Lane and Road Marking Annotation:
- Talent the exact line and road marking annotation methods by applying the quality precise road geometry understanding vehicle.
- Precise delivery of lanes and road barriers is most relevant for stable navigation and lanes keeping.
3D Object Detection and Annotation:
- Turn labelling to 3D: Annotation of objects after depth level is set.
- This factor is simply critical to provide capabilities for deployed systems such as LiDAR and radar, as well as to merge them for a more complete environment perception.
Annotating Challenging Scenarios:
- Explain the confrontation of difficult situations for example, severe weather, low-light areas and complicated urban scenes during the annotations of the process.
- Bearing the annotation of few examples in complex and versatile scenarios makes the model to be generalized perfectly and used safely in different circumstances.
Anomaly Detection Annotations:
- Create training programs to develop annotation techniques for detecting unusual occurrences or the circumstances that aren’t supposed to happen in the environment.
- Deviations can be of various types, such as an unusual road surface, some improper buildings, or an incorrect movement of vehicles.
Human-in-the-Loop Annotation:
- Human-in-the-loop annotation strategies include implementing them for instances where automatic annotation tools can have difficulties, hence the provision of accurate annotations.
- Employees’ detailed knowledge becomes a vital component of the process able to successfully address those cases which need individual treatment and for establishing precise results
Data Augmentation Strategies:
- Use the well-earning data augmentation methods to artificially increase the amount of the given dataset.
- Data augmentation serves to widen the territory of training and equipping the model by exposing it to larger data masses and a better representation of real-world instances.
Continuous Model Improvement:
- Setup a feedback mechanism for ongoing model fine-tuning with regard to the actual field accomplishment.
- Leverage real-world annotated data produced during the operation the machine learning model to facilitate autonomous driving-related updates for future road conditions.
Ethical Considerations and Bias Mitigation:
- Incorporate ethical aspects as part of the annotation procedure for the usability of autonomous vehicle perception system by elimination of any bias and obtain fairness.
And through this application of the deep learning technologies, autonomous vehicles can be expected to achieve a higher level of precision, robustness, and safety in their perceptual and decision-making systems. Consistent revisions and enhancements to the annotation procedure serve the purpose of staying in line with new autonomous vehicle technologies and continuous real-world challenges that concept follows.
To know more about Annotation support’s annotation services, please contact us at https://www.annotationsupport.com/contactus.php