Annotation labelling services are the key factors that have positively contributed to increased accuracy in image recognition as they provide high-quality datasets of labelled images for robust model training.
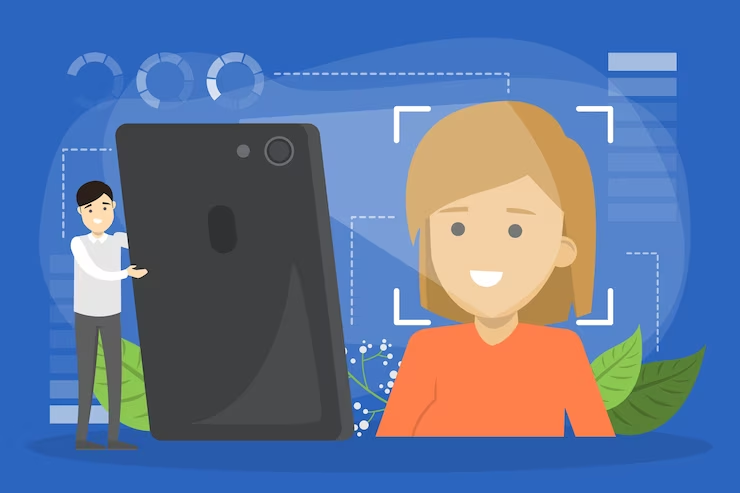
Here’s how annotation labelling services have contributed to improved accuracy:
Ground Truth Data: Annotation labelling services supply the models with correct data to train them on ground truth labels for images, making validation of the machine learning more effective. Through the specified placement of labels, with the use of bounding box annotations, semantic segmentation masks, or keypoints, annotation services provide the ground truth required for AI models to distinguish and classify objects or features, or any other data contained within the images.
Diverse and Representative Datasets: Annotation services are used for assembly of multi-faceted and the reflection of the diversity of datasets through image labelling from different resources, which include different views, lighting and backgrounds occlusions. Adjusting the AI models using multifaceted datasets enhances the robustness and generalization abilities of the system, which results in better performance in the real-life situations.
Fine-Grained Annotation: The services of annotation tagging necessarily have to have fine-grained annotation of images which give the possibility to identify and localize precisely the objects and regions of interest inside images. Methods like semantic segmentation and landmark annotation fix object boundaries which help undertake more descriptive learning and improved understanding of complicated visual scenes.
Quality Control and Assurance: Annotation is usually about the quality control that is necessary to guarantee the accuracy and entailment of the labelled data sets. Processes like multiple rounds of annotation, inter-annotator agreement exams, and quality assurance checks are some of the ways that we detect and fix mistakes in labelling. Hence, only top-quality data is used as a basis for AI algorithm training.
Semantic Understanding: Annotation providers speed up the process of training machine learning algorithms and also give them a higher semantic understanding of images content through labelling; e.g. some image data is labelled in such a manner that they understand what the concepts behind that image content are. It is this semantic concept apprehension that allows AI models to combine different scene and item variations and gradually leads to better object and image classification.
Adaptation to Specific Domains: Annotation services can be precise to selected domains or applications such that a formation of a dataset that is applicable for the use of distinct use cases starts. A related instance is where titters for medical imaging are developed with annotation services that carry labels needed by various tasks for example lesion detection or tumour segmentation to finally have better accuracy in medical image analysis applications.
Iterative Improvement: Annotation labelling services commit to an unending development, which is due to improvement not ceasing with labelled datasets refinement. Since the models are trained and deployed in real-world application, the performance and user inputs such as feedback would help to iteratively update and improve the original dataset, which in turn leads to further increases in model accuracy.
Indeed annotation labelling services have immensely taken the accuracy of image recognition to a higher level by offering credible labelled datasets that are large and diverse enough for the achievement of superior results to train and tune AI based models to reach top notch performance in different applications.
To know more about Annotation support’s annotation services , please contact us at https://www.annotationsupport.com/contactus.php