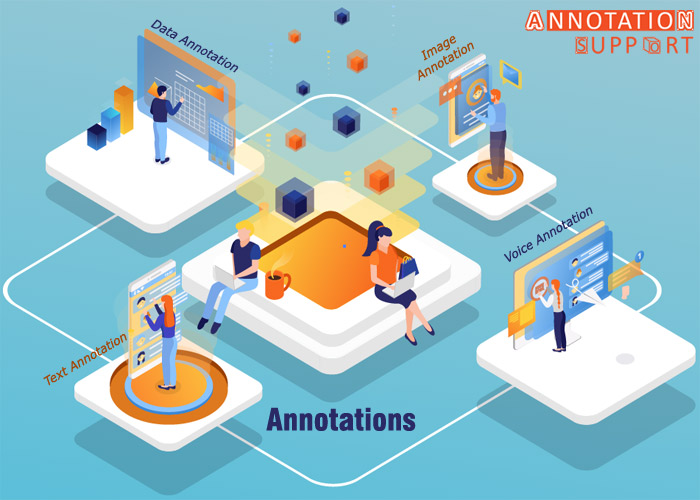
Computers cannot process visual information in the same way as the human brain. Computers must know what they are interpreting and provide context for decision-making. Data annotations establish these relationships. Labeling content such as text, audio, images, and video is a human-driven task that could be identified by machine learning models and used for prediction.
The practice of labeling data to show the outcome you want your machine learning model to predict is known as data annotation in machine learning. You’re marking up a dataset with the properties you want the machine learning system to learn to recognize by labeling, tagging, transcribing, or processing it. You want your model to be able to recognize those features on its own once it’s been deployed.
Data annotation is thus a vital and surprising achievement given the current rate of data generation.GM Insights forecasts that the global market for data annotation tools will grow nearly 30% annually over the next six years, especially in the automotive, retail, and healthcare sectors.
How are data annotations processed?
The data tagging process involves tagging photos, videos, audio, classification and textual information. It is primarily processed in machine learning to create datasets that enable machines to understand and act on input data.
Different Types of Annotations
1) Image Annotation
Such annotations are needed to annotate all types and formats of images for machine learning and Al model developments. The task of accomplishing annotation as per the client’s customized needs with turnaround time, affordable pricing while ensuring the data safely at each level and delivery at the best quality.
2) Video Annotation
Just like an image annotation,
video annotation is also used to make objects in videos detectable to machines through computer vision. The frame-by-frame annotation process is done to make the entire video labeled with a bounding box or semantic segmentation. From self-driving cars to track human activities, video annotation is used to train Al models.
3) Text Annotation
Provides multilingual metadata using text annotations for automated, high-quality image processing for machine learning and robotics. It’s done for natural language processing, which allows machines to read word text in different languages for accurate NLP in machine learning and artificial intelligence.
3) 3D Point Cloud Annotation
Performing annotation with point cloud data or LiDAR data. The process is primarily used to annotate road scenes for autonomous vehicle projects. The machine learning data will be used to build driverless cars.
4) Healthcare Annotation
It caters to the medical imagining data for Al training in the healthcare sector. It provides precisely annotated medical images like X-rays, MRI, CT scan, and Ultrasound for machine learning and Al model training through computer vision. Various types of medical Images are annotated with different techniques as per the requirements while ensuring the accuracy and quality of data sets to help Al and ML developers build a successful model at a low cost.
WHY do we need data annotations?
In computer vision education or pattern recognition solutions, people need to identify and describe some data, such as selecting pixels from an image that contains trees or road signs. With the help of these structured annotations, machines can learn to recognize these relationships better during testing, labeling, and manufacturing. Data annotation is a thriving field for artificial intelligence and machine learning, which has contributed significantly to the advancement of the world.