The path of data annotation is branched into many innovations which can lead to faster and more accurate work with better and efficient scaling features. Here are some key trends and innovations expected to shape the future of data annotation:
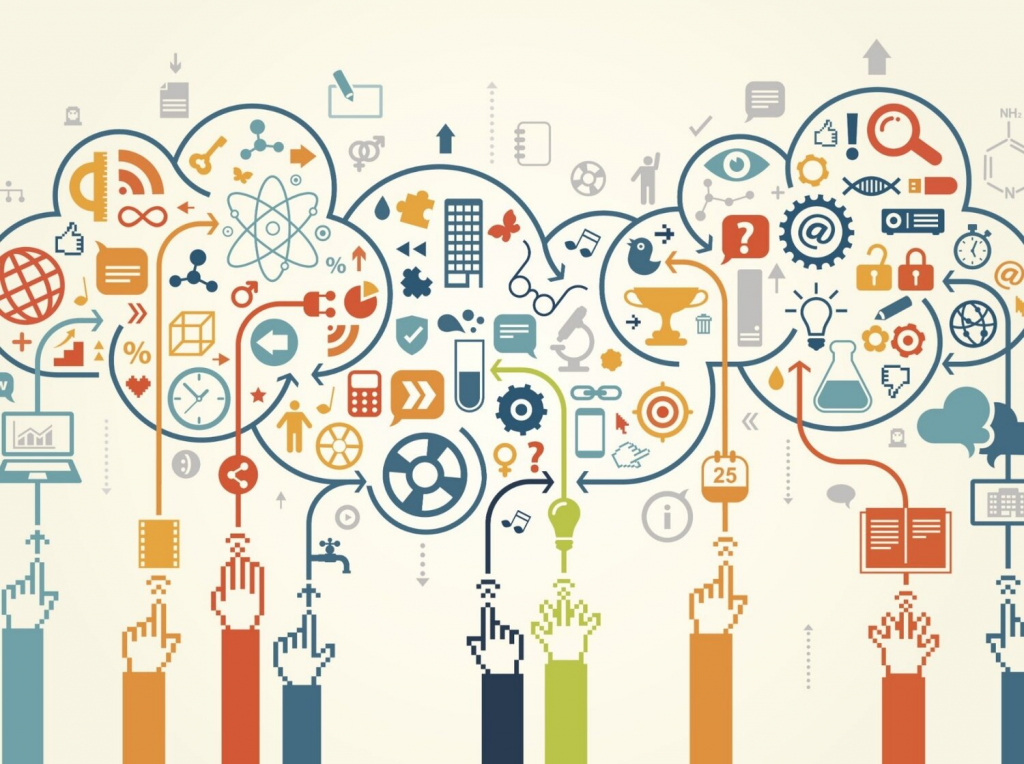
Automated Annotation Techniques: Automated or semi-automated annotation mechanisms based on developments in computer vision and natural language processing are becoming more prevalent. These methods in turn leverage AI algorithms to annotate data by themselves, which not just saves time and money on manual labelling but also accelerates the whole annotation process.
Active Learning and Human-in-the-Loop Annotation: The active learning algorithm, based on the human-in-the-loop toolkit, makes annotation procedure much more efficient. These methods proactively choose the sources of the most valuable information among annotated data, allowing the human experts whose knowledge is much needed exactly when it’s the most needed.
Semi-Supervised and Self-Supervised Learning: Partial supervisory approach and self-supervised learning decrease the necessity of full scale labelled data for learning. Through utilizing partially labelled or unlabelled data, these techniques are the source of more economical annotation strategy which at the same time, does not bottleneck model performance.
Multi-Modal Data Annotation: AI applications success rate is hugely dependent on the need to involve multiple data modalities such as images, texts, audio, and video, thus, the need for multi-modal data annotation services is higher than ever before. Through annotation labelling services, there will be an increase of abilities to deal with varied data types and will, in turn, help in development of a more comprehensive AI solutions.
Crowdsourcing and Collaborative Annotation Platforms: Collaborative tooling, crowdsourcing platforms combined with distributed annotators enable to handle labelling tasks efficiently altogether. Such platforms provide easy-to-scale annotation workflows, along with quality control mechanisms, and in-process collaboration among many people working together, allowing for the annotation of large-scale datasets.
Domain-Specific Annotation Expertise: Annotation labelling sector will be personalized in specific domains; this will give the ability to give out domain-customized expertise by all industries and application. Each domain is assigned with unique annotation services that is verified and targeted for particular cases.
Privacy-Preserving Annotation Techniques: As there is a drastic rise in data privacy and security issues, annotation labelling services will consider privacy-preserving practices that shall not compromise the confidentiality of sensitive data. For example, the privacy preserving technologies such as differential privacy and federated learning can be used to a shared annotation process while preserving the integrity of data.
Quality Assurance and Annotation Consistency: Innovations in the formula of recognized quality assurance methodologies and annotation consistency check mechanisms will guarantee the reliability and consistency of annotation datasets. Automated quality control measures, inter- annotator agreement metrics and feedback loops will be applied to keep the annotation quality at a high level.
Adaptation to Emerging Technologies: Knowledge in annotation services will be able to cope with emerging technologies with edge computing, IoT devices, and AR/VR systems being some of them. The latest technologies are changing the way data annotations are made and used in the modern world, aiming to find new solutions to old problems and, in this way, to upgrade the services.
Ethical and Bias Mitigation Considerations: The future of annotation labelling will devote much time in finding solutions to ethical questions and balancing the datasets with bias reduction. The utilization of ethical rules and bias-detection algorithms in the annotation process in a diversity-sensitive manner contributes to the guarantee of the fairness and inclusivity of AI systems.
Accordingly, the future in data annotation will feature the development of novel methods that make use of AI, automation, collaboration, and knowledge to move with the changing demands of the AI. Such developments will further spread annotation labelling services in various industries and also serve as the foundation to the development of more mature and responsible AI applications.
To know more about Annotation support’s data labelling services , please contact us at https://www.annotationsupport.com/contactus.php