Geospatial annotation services enable AI companies to create innovative solutions which benefit the sectors of agriculture together with forestry ,urban planning , transportation and environmental monitoring. AI models achieve high accuracy through precise marking of satellite imagery as well as aerial data and LiDAR point clouds which helps them understand spatial environments properly.
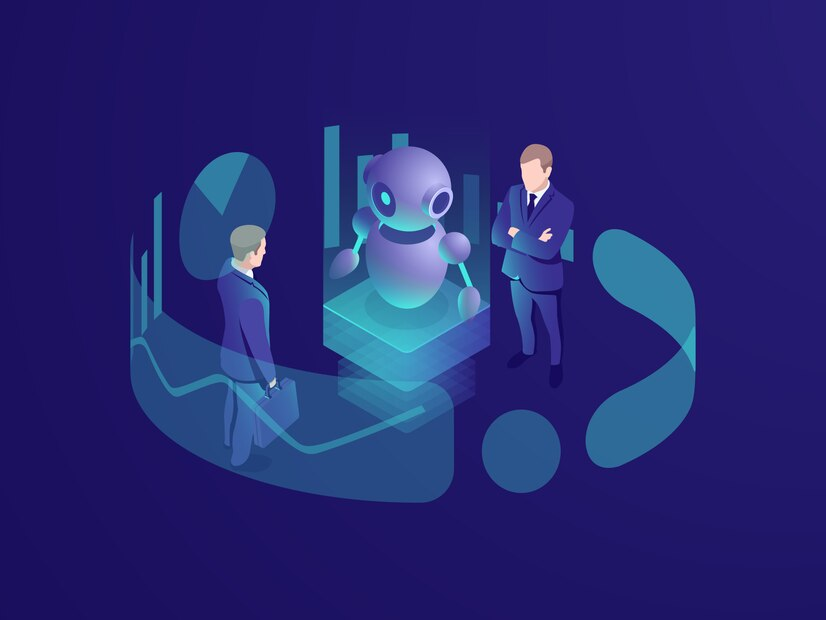
Geospatial annotation service providers have introduced various important technological advancements.
AI-Powered Precision Agriculture
The training of machine learning models for optimizing farming practices depends on data from AI firms which received contextual annotation from geospatial data.
- Drone images that receive annotations enable machines to predict yield production through assessment of plant development stages.
- Static AI models detect crop problems by analysing pictures sent to them.
- The implementation of annotated datasets leads to improved water and fertilizer distribution systems under the category of Smart Irrigation & Resource Management.
Urban Planning & Smart Cities
Geospatial annotation acts through the help of AI companies to enable urban environments to become more intelligent.
- The classification of land operated by automated systems utilizes aerial and satellite imagery.
- City traffic becomes more efficient through traffic and infrastructure monitoring using annotated road network information.
- AI systems analyse disaster-specific geospatial data sets that have been properly annotated to determine flood vulnerability together with infrastructure strength.
Forestry & Environmental Conservation
The process of geospatial annotation has become instrumental for modern environmental conservation practices in forestry operations.
- Deforestation along with illegal logging assessment becomes possible when AI systems process labelled satellite pictures that show changes in tree distribution.
- The observation of thermal imagery helps forecast dangerous wildfire regions before the fire expands across them.
- The companies that specialize in wildlife conservation track animal populations and monitor habitat changes with the help of geospatial data sets that underwent annotation.
Autonomous Vehicles & Logistics
Navigational accuracy for self-driving technology operates based on the use of geospatial annotation provided by companies in this sector.
- The processing of accurate maps depends on AI technology which analyses LiDAR and satellite data that humans have annotated previously.
- AI models acquire knowledge from classified geospatial data to detect road signs along with recognizing both traffic warning signs and pedestrians.
- The process of optimizing drone deliveries benefits from aerial images that have been expertly labelled and annotated.
Defence & Security Applications
The interpretation of geographical information has essential functions for both security operations and national defence tasks.
- Patriot Intelligence Services analyse photo-referenced satellite images to provide instant intelligence information.
- The analysis of unauthorized movements on terrain data by AI-powered drones takes place through border surveillance operations.
- Disaster Relief Operations – Geospatial AI supports search-and-rescue missions in affected areas.
The evolution of artificial intelligence together with automation will make geospatial annotation services more efficient while increasing scalability thus resulting in worldwide industry transformation.