Data annotation services help to greatly improve sports analytics by transforming raw sports data (images, videos, sensor data) into structured, labelled datasets that can be used for performance analysis, strategy formulation and decision making. All tokens come from annotated sports data and the combination of AI, machine learning and sports data allows teams, coaches and analysts to gain a greater depth of insight into player performance, game strategies and even audience engagement.
Here’s an in-depth exploration of how data annotation services enhance sports analytics:
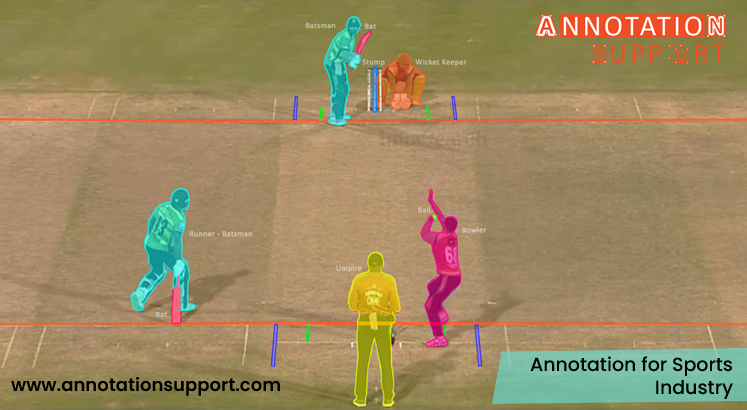
1. Player analysis and Performance tracking
Application: Sports data is annotated to track how players move, behave and act on the field to help coaches and analysts understand an individual and team performance.
Role of Data Annotation:
Pose Estimation: Key body points, such as the head, elbows, knees, are labelled in videos through data annotation services that serve as a reference to AI models to track player movement.
Event Tagging: This can include, but is not limited to, video footage identifying and labelling specific in game events such as passes, tackles, goals and turnovers through annotated video footage.
Outcome: This leads to actionable insight into player positioning, speed and efficiency which can help a coach as they look to optimize training regimens and playing strategies.
Example: On a soccer emulative video, annotated video data can track running speed, direction changes or possession times and match teams can adjust tactics or pay attention to fatigue.
2. Game Strategy and Tactical analysis
Application: Data annotation is used by sports teams to analyse tactical patterns from games, like formations, play tactics and opponents tendencies.
Role of Data Annotation:
Game Situation Labelling: Cast a problem into specific scenarios such as corner kicks, free throws or power play, and then an abstract can label that scenario so that AI models can recognize the patterns.
Zone Identification: Instead, spatial analysis of team formations and player positioning is possible through play zone annotations allowing annotators to label different zones on the field or court in which plays develop.
Outcome: Teams can use these insights to engineer counter strategies, identify weakness in an opponent’s game, or improve their thinking on game decisions.
Example: For example, in basketball annotated data helps identify key moments in defensive breakdowns during offensive plays.
3. Video Highlights, Generated Content
Application: To enable fans and analysts to have access to highlights, to compile performance metrics, or to view comprehensive game reviews automatically, videos of sports games are annotated and sports highlights, performance metrics, detailed game reviews are generated automatically.
Role of Data Annotation:
Highlight Tagging: Exciting or significant moments such as for example goals, touchdowns, dunks, penalty shots can be automatically compiled into highlight reels by the annotators who label them.
Key Player and Action Tagging: Annotators focus on specific actions by players, among them key passes, goals, assists and so on, turning the data on individual performance breakdowns.
Outcome: Because they are customizable, sports broadcasters and analysts can quickly create content specific to any game, and teams can review critical game moments with no manual intervention.
Example: Automatic creation of highlight reels featuring top plays, assists, goal scoring opportunities, etc., of football match from annotated game footage.
4. Health monitoring, Injury Prevention.
Application: Data annotation services can go to analyse player biomechanics and football motion behaviours in order to detect irregularities that may indicate the presence of injuries.
Role of Data Annotation:
Posture and Gait Annotation: With the help of AI systems, players’ postures, gait and biomechanics can be labelled, which allows tracking of deviations from the normal patterns.
Impact Analysis: Injury risk and impact severity on the actions are labelled by annotators by annotating instances of physical contact, falls, or collisions.
Outcome: Preventive measures can be replaced by teams and players may change training loads to prevent injuries and maximize recovery time.
Example: Annotating movement data in sports like tennis or basketball allow early injury detection such as signs of muscle strain and overuse injuries and early intervention.
5. The Fan Engagement and Experience Enhancement
Application: Interactive features, augmented reality (AR), or personalized sports content is created by leveraging annotated sports data.
Role of Data Annotation:
Fan Preferences: Fans would typically interact with moments or actions, big plays, star player highlights, dramatic game moments, and more, all of which are annotated by fans.
Content Customization: We use labelled data to provide personalized recommendations, in-game analytics, or augmented experiences as a part of an in game event (live game).
Outcome: This data can then be leveraged by sports organizations to provide more compelling and interactive fan experiences that can increase fan loyalty and retention.
Example: Powered by annotated data, real time analytics overlays in AR apps allow users to see player stats, speed, and positional data in real time, during a live game.
6. Officiating and Rule Enforcement
Application: A data annotation helps train AI systems that can help referees make real time decisions by identify rule violations and re-emphasize contentious moments.
Role of Data Annotation:
Foul Detection: In game footage, game fouls, offsides, or other rule violations are annotated by the ones and AI models then detect similar instances in real time.
Line Calls and Ball Tracking: Referees have Annotators to help them label ball trajectories and line boundaries to help make close call decisions.
Outcome: Through training with annotated data, AI systems can assist the referees to make quick, accurate decision and eliminate human errors.
Example: Data annotation in tennis helps AI know if a ball was in or out, allowing umpires’ decisions to be more accurate.
7. Predictive Analytics and Match Outcomes.
Application: AI systems use annotated historical sports data to make match outcomes, player performance, or fan engagement trend predictions.
Role of Data Annotation:
Historical Event Labelling: By training models, past events are annotated and past events like team formations, scoring patterns and so on are annotated to label to train the model in predictive analysis.
Performance Trend Analysis: Performance metrics achieve the label of repeated events over time, where AI then discovers performance patterns like player fatigue, scoring consistency, or team synergy.
Outcome: Predictive models of annotated data can let teams predict outcome of a game, optimize player performance, and even plan out future games.
Example: Annotating past matches with score and assist probability for each player, we can then predict which players will likely contribute towards future goals (knowing current game conditions) based on their past match performance.
Conclusion
In sports analytics, data annotation services are essential. These services enable AI and machine learning models to extract meaningful insights by giving accurate and detailed labels for sports data for improved performance tracking, better fan engagement and for aiding referees in decision making. With the evolution of sports analytics, the importance of data annotation as a foundation for innovation will continue to be a central pillar towards building the next best strategies for teams, athletes and sports organizations.
If you wish outsource sports annotation process please contact https://www.annotationsupport.com/contactus.php