Autonomous vehicles, one of the revolutionary technologies in the contemporary world, are set to drastically transform transportation. Deep at the center of these self-driving car(s) is an artificial intelligence engine which relies greatly on large datasets that are tagged correctly. Self-driving car systems necessarily require data annotation services, which refer to the process of labelling data. By enabling vehicles to understand and interpret their surroundings, data annotation has emerged as the backbone of autonomous driving technology.
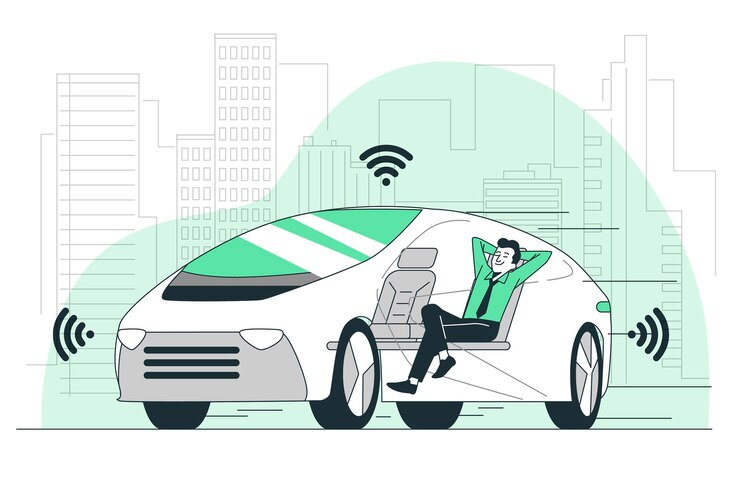
The Role of Data Annotation in Autonomous Vehicles
Perception in self-driving cars is achieved through various systems such as cameras, LiDAR – Light Detection and Ranging, radar and ultrasonic systems. These sensors produce a huge volume of raw data, which should be correctly analysed by AI of the vehicle to make necessary immediate decisions at the moment, including the detection of the obstacles on the way, recognition of traffic signs, and the forecast of the actions of the pedestrians on the crossroad.
Data annotation services enable this process by providing the following key capabilities:
Object Detection and Classification: They identify objects that are present in images and videos collected by the vehicle’s vision systems; these include but are not limited to; pedestrians, traffic signs, and other cars. It enables the AI system to effectively identify, categorise and then interact with an object in real time.
Semantic Segmentation: This means assigning each pixel of an image with a particular category (e. g., road, sidewalk, vehicle, etc.) so that it can be able to distinguish the various features of the surroundings accurately. Semantic segmentation is important for such tasks as lane detection and avoidance of the obstacles on the road.
Bounding Box and Polygon Annotation: The definition of the shape and position of objects in the image use bounding boxes and polygon. They assist the self-driving cars to estimate the scale and position of the objects in 3D space.
3D Point Cloud Annotation: LiDAR provides a point cloud that is a three-dimensional model of the environment, providing perceptive depth to self-driving cars. Annotators assist in the tagging of this 3D information enabling the vehicle to establish depth and object tracking in real-time as this is imperative for successful navigation in them.
Tracking and Predictive Behaviour Annotation: Vehicles have to navigate through environments that are dynamic that is why it cannot only detect objects, but rather predict their dynamics. By annotating movement trajectories of vehicles, pedestrians, and cyclists, artificial intelligence has a better understanding of the planning behaviour that follows and a better chance at making good decisions for safety’s sake.
Impact of Data Annotation on Autonomous Vehicle Development
The quality of annotated data is decisive for the function of the self-driving systems. High quality annotations, which include the checking and validation, make certain that the AI models are able to perform well under various scenario such as different road terrains, weather circumstances and in the urban or rural settings.
Some of the ways in which data annotation services are driving advancements in self-driving cars include:
Enhanced Safety: Annotation services also contribute to the quality of labelled data, to have a better perception of possible risks that AI will decide and act upon. This is regarded crucial in avoidance of cases of accidents and achieving better control of traffic in areas of high traffic density.
Accelerated AI Training: Teaching machines to learn as humans learn with perception intelligence necessitates a big data with carefully annotated data. Annotation services facilitate this process by generating high volumes of labelled data to support further machine learning optimization.
Adaptability across Geographies: Self driving vehicles need to be able to respond to traffic signs, signals and other traffic conditions existing globally. Data annotation services provide region-specific data that locates AI systems by identifying particular nation’s attributes like traffic signs or road markings.
Real-World Simulations and Testing: To build such environment replicas as well as to perform simulations self-driving algorithms require annotated data. Such tests can be performed in a safer way in such conditions as sudden movements from the pedestrians or adverse weather conditions.
Challenges in Data Annotation for Self-Driving Cars
Despite its critical role, data annotation for autonomous vehicles faces several challenges:
Scale and Complexity: Automated cars produce large volumes of data daily, not least during road trials. Manual annotation of this data at scale, specifically, for datasets such as LiDAR point clouds, can be highly time and resource-consuming and require skilled personnel.
Accuracy and Consistency: Hence it important to ensure that the annotations are correct and consistent since any mistake in the labelling process may lead to a wrong AI decision that may compromise on the safety of the vehicle.
Edge Cases: Some of the most difficult situations to annotate are: labelling paths that are seldom applied (for example, animals on the road, linked and rapid movements of pedestrians). These situations must be distinctively incorporated into training data to have an assurance that vehicles will respond to the irregularities.
Time and Cost: Manual annotation, particularly of 3D and video data, may be expensive and time consuming and hence may not be a feasible option. The requirement to strike a fine line between high quality annotations and speed is still a difficulty for autonomous vehicle organizations.
The Future of Mobility and Data Annotation
Year by year, self-driving technology remains to be a key aspect in developing autonomous vehicles, and the job of data annotation is an important part of this process. In the future, improvements in AI based annotation tools and methods of active learning could alleviate and decrease the dependency of manual labelling making this process cheaper and faster.
Moreover, as the presence of self-driving cars increases in the future to become an integral part of transportation networks, data annotation services would require broader to encompass novel mobility that will be developed, including drone delivery networks and self-driving public transit systems. As mobility goes more toward fully automated systems, acquiring techniques to label progressively complicated data sets will be crucial.
Conclusion
Self-driving car revolution is incomplete without data annotation services. These services being the primary input to allow machine learning models build an understanding of the world for mobility to thrive in the future. With the advent of more developed and widely used self-driving cars, the necessity of high-precision, quality annotation will remain higher in the future, which will define the transport system of the future as an object of science fiction.
If you wish to learn more about Annotation Support’s data labelling annotation services,
please contact our experts at https://www.annotationsupport.com/contactus.php