Basically, the growth and dynamics of warehousing is currently on the next phase where application of artificial intelligence (AI) and machine learning (ML) is dominating the progress of the warehousing business in the market. These innovations have been recognized as the following with image classification taking the limelight as one of the novel technologies that can significantly bring changes to the main functions of warehouse organizations. This AI based approach allows for better, faster and more effective handling of operations in a manner that forms a basis of an almost fully automated warehouse.
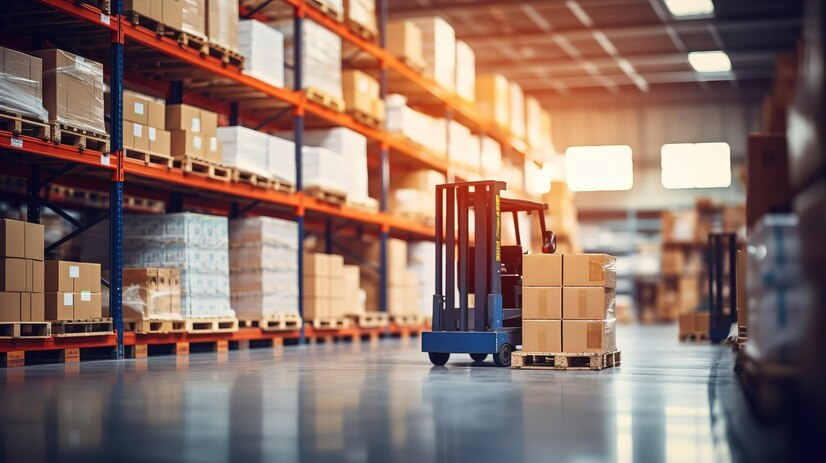
1. The Role of Image Classification in Warehousing
Image classification entails using machine learning to train the algorithm on a set of images so that the algorithm can identify objects for classification purposes. Through training these models with large-scale labelled pictures, it is possible to obtain models that can recognize products, packages, defects, and all those features that are crucial to warehousing. It can then be applied in different fields, not only the inventory control, but also the quality control.
2. Revolutionizing Inventory Tracking with Image Classification
In conventional methods of warehousing, inventory tracking entails the use of barcodes and RFID, together with manual scans. Although these techniques, they are slow, liable to human error, and expensive especially when applied in large-scales operations. Image classification addresses these challenges through its ability to:
- Automate identification: As a result of identifying products and packages visually, the need to scan barcodes or otherwise check the packages’ contents visually is eradicated. There are cameras throughout the warehouse that can take images of the products and the AI will sort them in real time so that there are fewer errors.
- Monitor inventory in real-time: Automatic image classification workstations are capable of identifying and tallying items on shelves or conveyors. This capability enables stock and product movement information to be determined in real-time thus enabling the determination of the status of inventories.
- Enable visual audits: The system can alert the system when there is a difference between inventory stocked digitally and physically, thus enabling the managers to do visual check of the stock without necessarily having to do a physical check.
3. Enhancing Quality Control with Image Classification
It can be noted that quality control of products plays a crucial role in warehouses especially in industries such as e-commerce, pharmaceuticals, and food industries, among others. Based on the previous research, quality checks have always been time-consuming and the results are normally based on the decision made by the inspector. Image classification is changing this by:
- Automating defect detection: With the help of AI, image classification systems can find out whether the products are damaged, labelled incorrectly or have defects. For example, a system can be designed to recognize scratches, dents or wrongly aligned labels so as to prevent poor quality products from being shipped.
- Speeding up inspection processes: AVI is also preferred since it provides faster check than manual inspection thus it has better throughput. With in-line inspection, the system only takes a short time for scanning for defects instead of waiting to identify the problems and then subjecting them to quality control to ensure that products are checked then released and dispatched in good time.
- Ensuring consistency: Image classification systems do not exhibit fatigue and variation during the various inspections that human inspectors are bound to show and hence, lead to increased variability in the quality control process.
4. Advanced Techniques in Image Classification for Warehousing
To maximize the impact of image classification in warehouses, advanced techniques are being developed to tackle the unique challenges of a dynamic environment:
- Convolutional Neural Networks (CNNs): CNNs are the fundamental building blocks of the images classification in AI. These networks are mainly intended to be applicable to image identification and are very efficient in use when it comes to tasks such as object recognition or even defect detection. Over the years, CNNs are finding application in the warehouses to support the autonomous working of warehouses.
- Transfer Learning: This unlike training the model from scratch enables the models trained on one dataset to be trained further using the other set specific to warehouses. This not only saves time and resources required for training but at the same time improving the accuracy.
- Edge computing: In real-time image classification, edge computing works by providing the processing power at the device level which minimises the distance to the edge. This cuts time, which means that the requirement of tracking inventory or inspecting part quality occurs instantly without relying on other servers which may be far apart.
5. Key Benefits of Image Classification in Warehousing
The integration of image classification offers significant benefits to warehouses looking to modernize their operations:
- Increased operational efficiency: Certainly, image classification systems save time down by doing away with manual errors facilitated by inventory tracking and quality control.
- Greater accuracy: Through the use of AI in image analysis, chances of wrong identification of a product as well as failure to detect defects are reduced and this positively affects the accuracy of the inventory as well as quality of the products.
- Scalability: Through image classification, expansion of warehouses without manual help is possible and hence only this method breaks the shackles of expansion.
- Real-time decision-making: Real time image analytics also provides improved shelf edge and stock management, order fulfilment confirmation and improved quality of offered products thus improving response time in warehouses.
6. Challenges and Considerations
While the potential of image classification in warehousing is vast, there are several challenges that need to be addressed:
- Data requirements: Generally, for the image classification models to give out the right results they have to be trained on large data sets or image data sets that are labelled. It is labour demanding and may require one to purchase primary data and spend a lot of time sifting through it so as to make notes out of it.
- Handling variability: Wholesalers encounter variable or numerous product types, light exposures, and packaging materials in their warehouses. Variability is inherent in images and traffic signs which means that image classification models need to be designed for this to avoid a reduction in their accuracy.
- Integration with existing systems: To optimize the benefits it is crucial for the image classification systems to be perfectly compatible with other WMS and other elements of warehouse, including robotic systems, conveyor systems, and databases containing the information about the inventories.
7. The Future Outlook: Fully Autonomous Warehouse
At the same time looking forward to it there are definite prospects for the development of image classification in warehouses. The convergence of AI, computer vision, and robotics will drive the development of fully autonomous warehouses, where robots powered by image classification and machine learning perform all major operations:
- Robotic pickers: Picture with automatic cameras and proper picture recognition algorithms and software, the robots will be able to pick products on their own without any human intervention.
- Autonomous quality control: Finally, vision system equipped robotic arms will conduct automated inspection in an endeavour to satisfy the company’s assurance that every product is inspected for conforming to the set standards before dispatch.
- Smart inventory systems: Real time image classification will make the inventory systems to be able to identify low stock levels and activate restocking process with no interference from human beings thus enhancing the performance of the entire warehouse.
Conclusion
With developing technologies of AI and machine learning, new innovation of image classification becomes more imperative to warehousing as it changes both the ways of inventory and quality check. The implementation of image classification enhances these processes’ accuracy and efficiency while laying the foundation for automated warehousing systems in the future. It can therefore be said that, through adoption of this technology in their businesses, organizations are able to improve on their performance, whilst at the same time, working on their costs and beating their competition within the emergent environment that is characterized by high and elevated velocity.