If machine learning and artificial intelligence models need to learn patterns and make predictions, then they need data annotation services to get their data present in a manner they understand. There are different kinds of data annotation services available that serve different applications, and they have different characteristics, as well as their own methods of conduct. Here’s an in-depth look at the main types of data annotation services support particular machine learning and AI tasks.
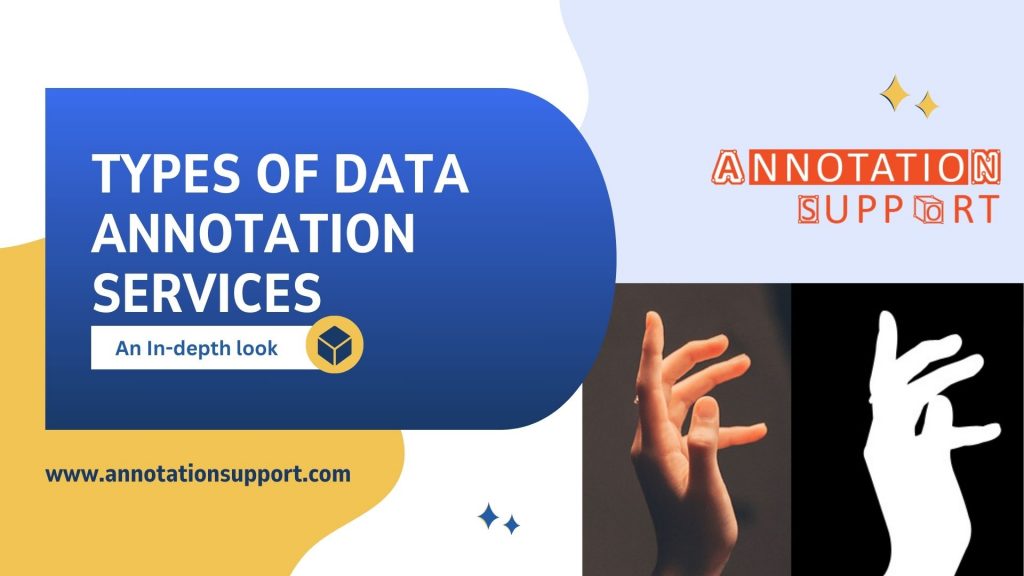
1. Image and Video Annotation
Bounding Boxes: Bounding boxes are rectangles, drawn around objects to tell where they are. In applications such as autonomous driving and security surveillance, where objects must be located (cars or people), this is a natural method of approach.
Polygon Annotation: Irregularly shaped objects that won’t fit in a rectangle are best suited to polygon annotation. Applications where boundary detection is of paramount importance, including medical imaging and autonomous drones, use this method.
Semantic Segmentation: That’s simply labelling each pixel in the image on it with a class label (e.g. “road”, “vehicle” or “pedestrian”). Pixel level accuracy is required in the field, such as in autonomous driving and environmental monitoring, where semantic segmentation is very popular.
Instance Segmentation: Instance segmentation is different from semantic segmentation in the fact that instance segmentation labels each instance of the same class, while semantic segmentation labels only the class. At the same time, it’s important because many applications want to distinguish between the same object, like how you might count individual trees or animals.
Video Annotation: For the video data, annotations are done frame level wise indicating the movement and time changes. In action recognition, motion tracking, and behavior analysis, this is useful, applications include sports, surveillance, and robotics.
Named Entity Recognition (NER): Entities are the things that make up text (NAMES, ORGANISATIONS, DATES, etc) and NER identifies and categorizes them. This is very useful in natural language processing (NLP) like sentiment analysis, customers support and information retrieval.
Sentiment Annotation: In sentiment annotation, one tags text containing emotional tone (positive, neutral, negative). This type is very commonly used for social media monitoring, customer feedback analysis and brand reputation management.
Linguistic Annotation: Such includes syntax, grammar, as well as part of speech tagging. These annotations help the language models and chatbots understand how the sentences are structured and what might be the context behind it.
Entity Linking: From NER, Entity linking goes further by linking to a DB or a knowledge graph. The most exciting application of CF is to improve the relevance of the retrieved information in recommendation systems, search engines, answer question systems, etc.
Speech Recognition Annotation: In speech recognition, a model is trained in conversing audio to text where transcriptions of spoken language are produced and provided. But much of the use comes from in virtual assistants, transcription services and automated customer support.
Speaker Identification and Diarization: Speaker identification tags specific speakers to an audio file while the diarization marker is a section of audio for which a specific speaker is tagged. In multi speaker environments like meetings, call centres and voice authentication, these annotations are crucial.
Sentiment and Intent Annotation: And we have these annotations that tell you what the tone or intent of the spoken words are — this is very important for conversational AI and customer service analytics.
Audio Classification and Tagging: The sounds are labelled with category (e.g. ‘laughter’, ‘applause’, ‘alarm’) in training to models that have applications in security, entertainment, and environmental monitoring.
3D Bounding Boxes: Like in 2D bounding boxes, 3D bounding boxes are objects that encapsulate 3D objects. Object detection in LiDAR data is an indispensable form of annotation in autonomous driving.
Semantic and Instance Segmentation: This is point cloud data segmentation, which adds labels to individual points in a 3D space – based on what they are, e.g. an object – making it perfect for identifying particular structures in very complex environments, like urban planning or even construction.
Trajectory and Path Annotation: Annotation in this sense is about tracking an object’s movement through a 3D space over time. In robotics and drone navigation for example, understanding movement paths is required and commonplace.
5. Human Activity Recognition (HAR) Annotation
Pose Estimation: Key body parts (for example arms, legs and head) are labelled to describe body posture in pose estimation annotations. The fitness, motion analysis and healthcare applications utilize this annotation type.
Behavioral labelling: Classifying things like licking, walking, running, sitting, or fetching the cat is what models can do when human activities are annotated. Sports analysis, smart home applications, elderly care monitoring, or other things are the things this is commonly used for.
Sequential Frame labelling: Each frame of videos is labelled to monitor the continuous activities in time. Applications in security, retail and in behavioural research can make use of it.
Conclusion
Different data annotation types solve different needs for particular purposes, thus the need to choose a type of data annotation appropriate to the use case of your application. However, high quality data annotation services for these types of data enable us to accurately and efficiently train machine learning and AI models and move our technology forward in domains like computer vision, NLP and autonomous systems.
Interested to get high quality and data secured annotation services ,contact us at https://www.annotationsupport.com/contactus.php