Medical imaging and diagnosis in the healthcare domain are driven by advances made on the data annotation among others to enhance accuracy. Machine learning models require accurately labelled data sets to recognize the patterns, detect abnormalities and support an effective diagnosis by medical specialists.
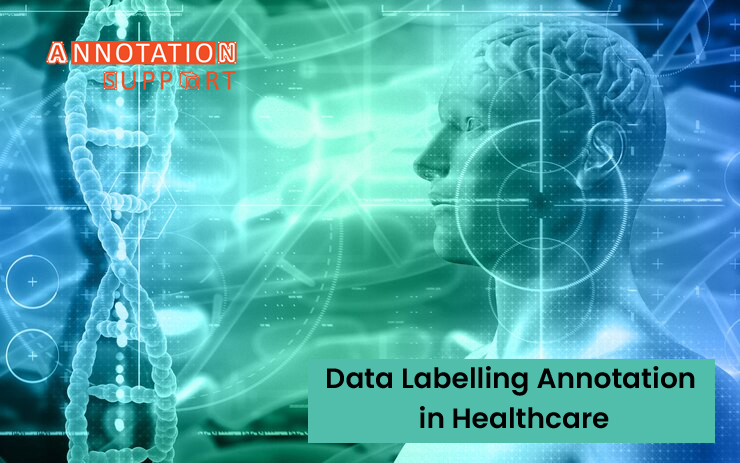
Here are some key aspects of data labelling annotation in healthcare:
Image Annotation for Medical Imaging:
Bounding Boxes: The bounding boxes depict the annotation of regions of interest (ROI) in medical images; this allows the algorithms to focus on a particular area, such as tumours or abnormalities during their training.
Segmentation: Annotation at the pixel level such as semantic or instance segmentation provides a better characterization of boundaries for the structures leading to more accurate identification.
Annotation of Pathological Features:
Lesion Annotation: It is very critical to identify and annotate the lesions, tumours or any abnormalities on medical images for training classification models which distinguish healthy from diseased tissue.
Anatomical Landmarks: Labelling anatomical landmarks leads to the proper localization and orientation, thus facilitating the correct analysis as well as interpretation of medical images.
Multi-Modality Data Labelling:
Integration of Various Imaging Modalities: Use of data labelled by diverse imaging modalities including X-rays, MRIs CT scans and ultrasound gives the models the capability to generalize beyond different medical images in which they become versatile.
Clinical Data Annotation:
Electronic Health Records (EHR) Annotation: The addition of clinical information from electronic health records to the medical images provides a lot more context for diagnosis and treatment decisions.
Patient History Annotation: Annotation of the relevant patient history information such as demographics, previous comorbidities and also treatment regimens may help to better comprehend the case.
Quality Control and Validation:
Expert Review: The inclusion of healthcare providers in the annotation process serves to guarantee that accurate and reliable labelled data is being produced.
Iterative Feedback: The refinement of annotations is enabled by the continuous feedback loops between the annotators and also domain experts, which ultimately helps in producing quality labelled data.
Addressing Class Imbalance and Bias:
Balancing Datasets: Betting on an unbiased distribution of the classes present in a dataset allows for a lot more accurate diagnosis of both common and also rare cases.
Ethical Considerations: Fair and unbiased health applications require a lot of data collection, annotation, and also model training to mitigate any potential bias.
Data Security and Privacy:
HIPAA Compliance: Compliance with HIPAA regulations or any similar rules is very important to protect the patient’s privacy and also ensure data safety.
Anonymization: Elimination and encryption of the PII on medical images plus related information helps address the privacy issues.
Continuous Learning and Model Improvement:
Feedback Mechanisms: Feedback collection mechanisms from healthcare providers on the performance of the model in real world use cases enable continuous improvement and refinement.
Therefore, high-quality data labelling annotations in healthcare are very crucial to the establishment and application of the appropriate machine learning models with sufficient accuracy during medical imaging and also diagnosis. The system calls for the collaboration of the domain experts, data annotators and also technology experts to develop labelled datasets that are used in improving healthcare technologies.
To know more about Annotation support’s data annotation services, please contact us at https://www.annotationsupport.com/contactus.php