Artificial intelligence systems enabled integration with manufacturing operations to boost three fundamental areas: efficiency together with accuracy and automation capabilities. AI systems require constant human supervision to improve their performance thus human-in-the-loop (HITL) annotation emerged as a solution. Utilizing this method human operators work alongside machine learning models to deliver superior process accuracy and lessening errors during manufacturing.
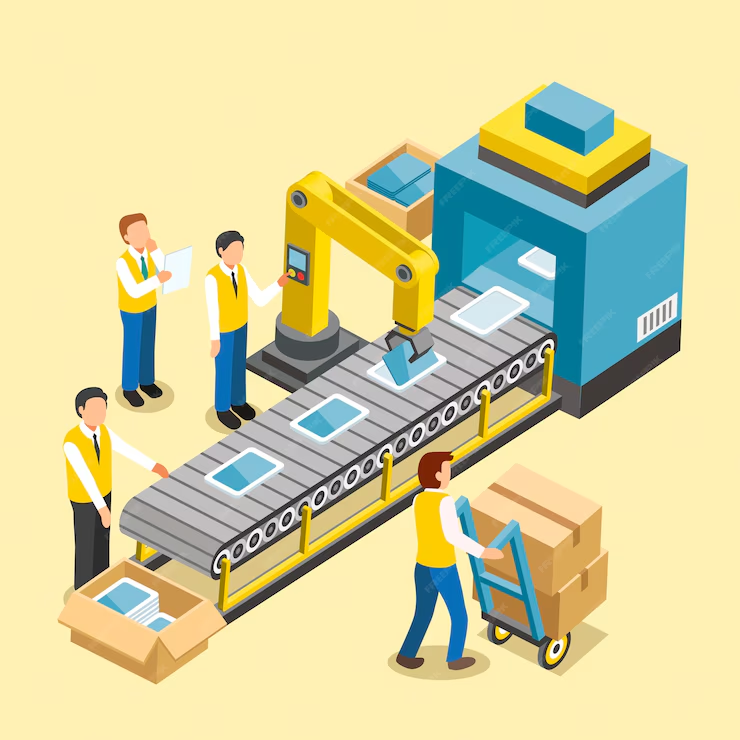
Understanding Human-in-the-Loop Annotation in Manufacturing
The method of Human-in-the-Loop annotation brings human evaluators into a continuous cycle that checks and modifies the automatic data annotation outputs from AI systems. Through an ongoing feedback system AI systems obtain human expertise to achieve stronger performance in handling advanced manufacturing situations.
Key Benefits of HITL Annotation in Manufacturing
a. Enhanced Quality Control
- The detection work of AI models for manufacturing defects reaches human annotators who perform verification of uncertain products.
- The higher accuracy achieved in detecting defects depends on the process of ensuring proper identification of cracks alongside misalignments and inconsistencies.
b. Improved Predictive Maintenance
- The combination of AI predictive maintenance systems detects abnormal activities from equipment operating performance.
- Human operators examine uncertain situations to protect the system from generating fake alerts and maximize its availability.
c. Increased Adaptability in Production Lines
- AI models which receive HITL annotation training become able to handle changes in raw materials together with product designs and environmental conditions.
- People assisting AI systems can adjust their capabilities to handle novel system deviations through feedback.
d. Optimized Supply Chain and Logistics
- The management of inventory through AI systems collaborates with humans who inspect AI-generated data for accuracy issues in supply chain logistics operations.
- Operation efficiency improves through this collaborative effort which also prevents disruptions in the process.
Applications of HITL Annotation in Manufacturing
a. Automated Visual Inspection
- AI models evaluate images of products to detect particular flaws.
- The collected data passes through human reviewers who check AI results against actual predictions and supply feedback for improvement.
b. Robotics and Automation
- Robotic arms become more efficient in manufacturing assembly procedures when AI technology is applied.
- Machine experts enhance AI decisions regarding complicated operations that need exact precision.
c. Smart Manufacturing Systems
- The operational monitoring system powered by AI leads to maximum resource management along with enhanced energy utilization during manufacturing.
- The implementation of human operators maintains AI models to achieve operational objectives.
Conclusion
Manufacturing adopts human-in-the-loop annotation to produce results that unite artificial intelligence efficiency with human cognitive abilities. The collaborative system between artificial intelligence and human operators enhances manufacturing quality while simulating equipment maintenance needs and giving production flexibility for systems that become more efficient and resilient. The continuing development of AI technology depends on HITL annotation to maintain precision along with reliability and efficiency across modern manufacturing establishments.